共计 2816 个字符,预计需要花费 8 分钟才能阅读完成。
提醒:本文最后更新于 2024-08-30 15:00,文中所关联的信息可能已发生改变,请知悉!
工作概述
思路
- 对于每个 2 * 2 的格子计算最大差异度 $d_i$
- 对于每个 $33$ 的区域来说,都可以分出左上,右上,左下,右下,中间五个 $22$ 格子(四个大格子,一个小格子),并将五个最大差异度全排列
- 找出 $max|d_i - d_j|$
- 从此处分为两组
- 若中间格子属于个数少的一组,则存在噪声,且噪声为重合点
代码示例
# utf-8
# python3.8
# note20211229
import cv2 as cv
import numpy as np
def map_position(a):
if a == 1:
return 3
elif a == 3:
return 1
elif a == 2:
return 4
elif a == 4:
return 2
else:
return 0
# 获取差异度
def get_differ_degree(array):
return max(array) - min(array)
def check_noise(_4x4):
position = ['null', 'leftup', 'rightup', 'rightduwn', 'leftduwn']
# 中心格子
d0 = get_differ_degree([_4x4[1][1], _4x4[1][2], _4x4[2][2], _4x4[2][1]])
# 左上
d1 = get_differ_degree([_4x4[0][0], _4x4[0][2], _4x4[2][2], _4x4[2][0]])
# 右上
d2 = get_differ_degree([_4x4[0][1], _4x4[0][3], _4x4[2][3], _4x4[2][1]])
# 右下
d3 = get_differ_degree([_4x4[1][1], _4x4[1][3], _4x4[3][3], _4x4[3][1]])
# 左下
d4 = get_differ_degree([_4x4[1][0], _4x4[1][2], _4x4[3][2], _4x4[3][0]])
differ_degree = [d0, d1, d2, d3, d4]
sorted_dd = sorted(differ_degree, reverse=False) # 升序
adjacent_differ_from_sorted_dd = [sorted_dd[1] - sorted_dd[0], sorted_dd[2] - sorted_dd[1], sorted_dd[3] - sorted_dd[2], sorted_dd[4] - sorted_dd[3]]
if max(adjacent_differ_from_sorted_dd) < 5:
return position[0]
else:
max_index = adjacent_differ_from_sorted_dd.index(max(adjacent_differ_from_sorted_dd))
if max_index == 1:
if sorted_dd[0] == d0:
return position[map_position(differ_degree.index(sorted_dd[1]))]
elif sorted_dd[1] == d0:
return position[map_position(differ_degree.index(sorted_dd[0]))]
elif max_index == 2:
if sorted_dd[3] == d0:
return position[map_position(differ_degree.index(sorted_dd[4]))]
elif sorted_dd[4] == d0:
return position[map_position(differ_degree.index(sorted_dd[3]))]
else:
return position[0]
def denoise(channel):
row, col = channel.shape
flag = np.zeros((row, col))
for i in range(0, row - 3):
for j in range(0, col - 3):
# 提取 4x4 的单元像素格
each_4x4_in_channel = np.zeros((4, 4))
for m in range(4):
for n in range(4):
each_4x4_in_channel[m][n] = channel[i + m][j + n]
# check noise
# position = ['null', 'leftup', 'rightup', 'rightduwn', 'leftduwn']
noise_position = check_noise(each_4x4_in_channel)
if noise_position == 'leftup':
flag[i + 1][j + 1] = 1
elif noise_position == 'rightup':
flag[i + 1][j + 2] = 1
elif noise_position == 'rightduwn':
flag[i + 2][j + 2] = 1
elif noise_position == 'leftduwn':
flag[i + 2][j + 1] = 1
for i in range(row):
for j in range(col):
if flag[i][j] == 1:
channel[i][j] = 0
return channel
def main(noise, new):
"""
:param noise: 原始图片路径
:param new: 噪声图片路径
:return: null
"""
img_noise = cv.imread(noise, 1)
b, g, r = cv.split(img_noise)
"""
b_row, b_col = b.shape
fp = open('b_o.csv', 'w')
for i in range(b_row):
for j in range(b_col):
print(b[i][j], file=fp, end='')
print(",", file=fp, end='')
print("", file=fp)
"""
b = denoise(b)
g = denoise(g)
r = denoise(r)
img_new = cv.merge((b, g, r))
cv.imwrite(new, img_new)
if __name__ == '__main__':
noise_addr = 'img_noise.png'
new_addr = 'img_new.png'
main(noise_addr, new_addr)
效果
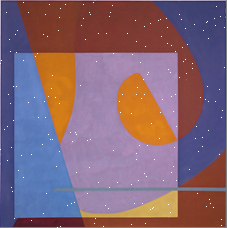
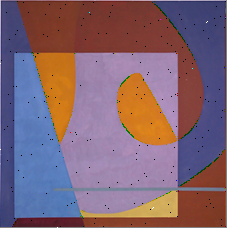
正文完